Oct 30, 2020
5 minute read
Topic
Quants must speak ESG
Quantitative approaches provide speed, scalability, and cost savings, which can help process the deluge of ESG data, says Habib Moudachirou.
"Darkest Quant Fears Ring True in $1 Trillion World of Smart Beta"1 , "Flash Crash — the trading savant who crashed the US stock market"2 , "Quant investing: The dangers of the Black Box"3 , "Goldman Sachs’ lessons from the ‘quant quake’"4 … Those headlines are, in essence, a reflection of the chants we have been hearing for years, coming from the bleachers of the financial markets’ arena, articles, podcasts, social media posts, etc.
Mindful of the natural tendency of headlines to overly focus on bad news, I often must methodically demystify those statements. The colossal amount of data available today for consumption, generally helps me make a case. In addition, one can even argue that the mounting demand for integration of sustainability in investment processes makes it a no-brainer.
Quant – today’s financial markets bogeyman; myth or reality?
With the intent of sparing the reader a history lecture on quantitative investing, one can simply define quantitative finance as the use of mathematic models to seek to achieve a defined investment outcome.
The historical focus in finance was on managing risk, measured by volatility. Quantitative finance can be traced back to the seventeenth century when merchants were rudimentarily trading ‘options’ to protect themselves. Subsequent versions, extensions and alternatives were later developed, all the way to the Fama-French factor model work and its later developments.
The need for speed in trade execution in the early 2000s, in conjunction with the ever-growing computing power of machines, created a new breed of investors or traders relying on computer programmes to automatically execute algorithmic strategies in a systematic way (e.g. high frequency trading). Speed gave them an edge and they became the poster child of quantitative finance, despite being only one of its applications.
1 Bloomberg, 2020
2 Financial Times, 2020
3 Refinitiv Perspectives, 2018
4 Financial Times, 2017
In 2009, during a meeting with a large North American pension plan, while explaining our then-global tactical asset allocation model, I vividly remember that moment when it dawned on me that, what I was saying was not what they were hearing. Until then, my proud insistence on the quant nature of our process has been predicated on the fact that our strategies were using mathematic models to inform and guide the investment decision-making process. However, repeated questions from the investors made me realise that they actually perceived quantitative investing as a strictly computer-driven process.
That assumption drove countless press articles and financial blogs to increasingly disparage quantitative finance. It was made a scapegoat of the 2008 great recession because of the (mis)use of complex toxic credit derivatives; it is also often blamed for market dysfunctions, and illusory promises of returns relying on convenient back-tests, diversification and mean-reversion models.
Nevertheless, we cannot deny that there has been an explosion of data, both structured and unstructured (see chart below), accessible to managers. As of 2018, the world was producing 2.5 quintillion 5 bytes of data every day, and this shows no sign of slowing down.
Furthermore, the financial industry is the largest consumer of data, and therefore needs to process it efficiently. Quantitative finance may have its critics, but it allows the harnessing and processing of a massive amount of data, which is put at the disposal of asset managers.
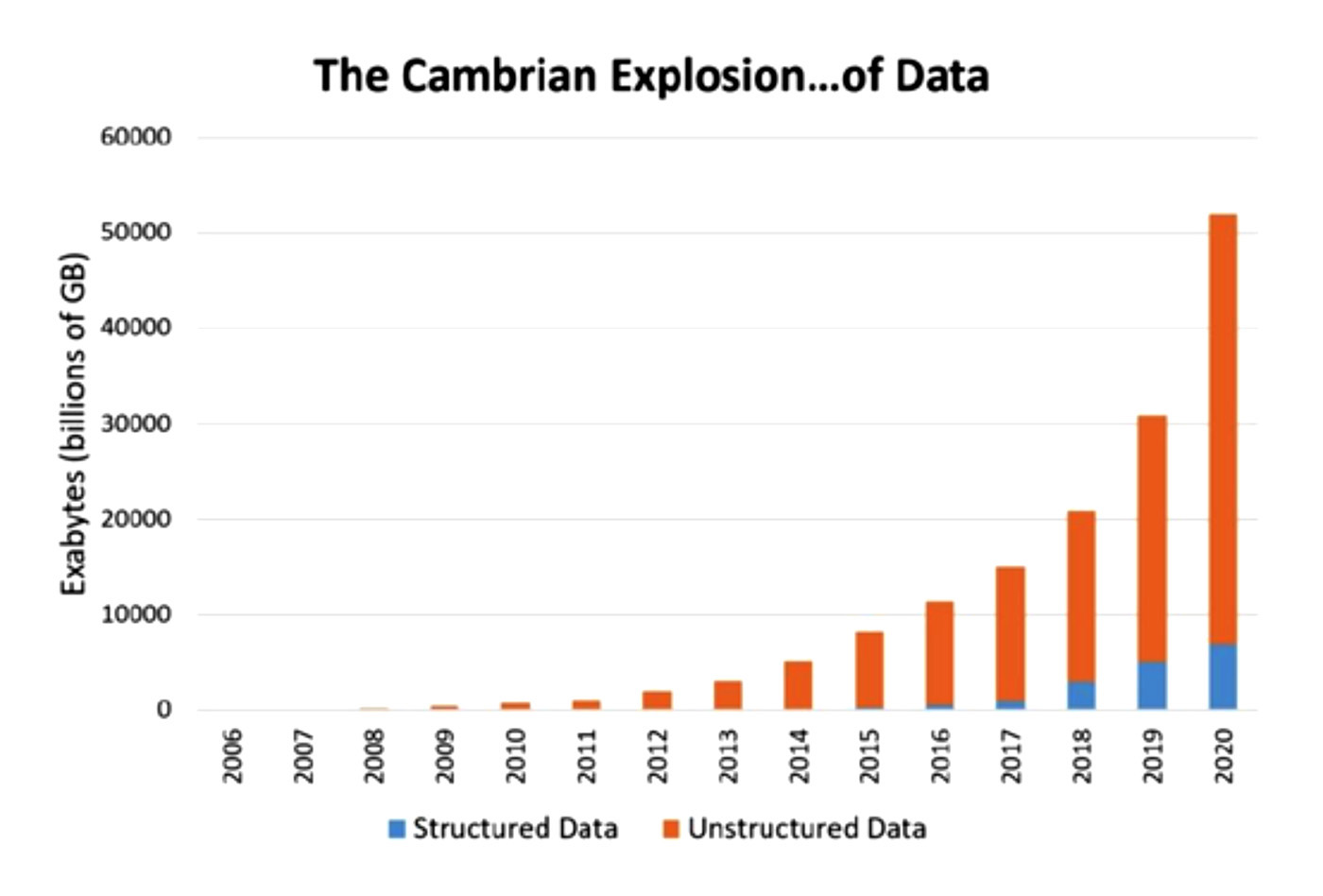
Source: www.eetimes.com
5 Forbes, Domo Business Cloud
Sustainability, the talk, the walk, and the boom
In the last couple of decades, the financial industry gradually embraced environmental, social, and corporate governance (ESG) matters as an opportunity to respond to investors’ growing demand, and to get ahead of future costly risks and negative externalities.
Sustainable investing has evolved over the years and took various forms, from socially responsible investing to impact investing. It also went from the marketing ‘insert’ status to a genuine effort to be integrated in investment processes in order to gain a performance edge or mitigate risk or fulfil regulatory requirements.
Without dwelling too much on the lexicon, sustainable, responsible and impact investing consider ESG criteria to generate long-term competitive financial returns and positive societal impact. The motivations are manyfold, from aligning with asset owner’s values, mitigating new forms of risk, to improving performance.
The total US-domiciled assets under management using sustainable investment strategies grew from $8.7 trillion at the start of 2016 to $12 trillion at the start of 2018, representing a 38% increase over 2 years 6. At the global level, the value of ESG-driven assets has almost doubled over four years, and more than tripled over eight years, to $40.5 trillion in 2020 7. This represents 45% — or close to one in two dollars — of the $89 trillion 8 in global assets under management. The growth rate in ESG investing remains steady, forming a “mainstreaming” trend.
Alongside the asset boom, dozens of data providers mushroomed, with offerings of research products and scoring methodologies destined to help asset managers and asset owners incorporate ESG. As a result, there is today an abundance of sustainability data, bent on measuring, and helping improve, the state of ESG issues.
The financial industry is an avid user of those metrics, with the goal of aligning investors’ values with investment processes, and potentially creating a dual positive impact. However, the overload of offers comes with a large dispersion of the ratings and scores of companies, as there is no unified nor universally agreed methodology.
The challenge, then, for any asset manager is how to select ESG data providers who will complement their investment processes and other financial analytics – a daunting task that is only a start. As a matter of fact, portfolio managers must have a view that helps them form investment decisions; that view encompasses not only a large set of data, but also experience navigating the markets, and conviction; for that reason they still need to develop a proprietary approach to fully express their view, in addition to the use of data/research providers.
The growing demand for integrating ESG considerations into investments must be reconciled with the deluge of data, which makes a compelling case for quantitative finance because capacity and speed are key; those are two archetypical features of quantitative methods.
6 US SIF Foundation Trend Report, 2018
7 Pensions & Investments, 2020
8 BCG Global Asset Management Market 2020 Report
Quant must speak ESG
The theoretical frameworks and the quantitative methods are constantly evolving and breaking new ground. For instance, regressions and time-series analyses were still heavily used at the turn of the millennium for their predictive power, and they were progressively complemented with a new generation of data science techniques that came out with the advent of big data 9 after the mid-2000s.
Nowadays, artificial intelligence (AI), machine learning and natural language processing (NLP) are among the disciplines and techniques that have become available to researchers and practitioners to sustain the exponential growth of data.
The financial industry has also evolved, evidenced by how sought-after quantitative developers and data scientists are now, a bit like traders and sales people who were similarly in demand 10 years ago. The large quantities and numerous types of ESG data create a challenge for portfolio managers, who must assess, untangle, and verify the data before consuming it.
They often must work with unstructured datasets in multiple formats (e.g. text files, filled forms, incident reports, audio, video) with coverage gaps, or that need mapping between the underlying dataset and the company being analysed. This requires advanced statistical techniques, such as neural-networks and support machines, which indeed pertain to the quant world.
Also, the handling of some of the new data, originating from alternative sources, naturally commands a quantitative approach. That category of data, termed ‘alternative data’, also comes in large amounts, including ESG data from information collected from individuals (or scanned) on social media, product and company reviews, blog content, satellite or drone imagery used to evaluate pollution emission quantity and intensity. Those require extensive additional processing if they are to be useful in a model.
Lastly, quantitative investing has an advantage over fundamental approaches in the areas of portfolio construction. In fact, the alignment with an asset owner’s sustainability values necessitates ongoing measurements, customisation, and more granular ex-post analysis.
A quantitative approach enables portfolios to be tailored to investors’ wishes in a systematic manner. Absent a universal measurement framework, prevalent ones are used, for instance, to measure the carbon footprint of a portfolio or the portfolio’s alignment with the UN Sustainable Development Goals 10 (SDG).
With greater scrutiny on the sustainability dimension in portfolios, ESG integration will compel more transparency from asset managers, as opposed to the usual “black box”, a typical criticism of quantitative models. And besides transparency, quantitative approaches provide speed of data processing, scalability, and cost savings.
9 Big data refers to datasets – structured and unstructured – so voluminous that traditional data processing software are unable to manage them. They require a set of techniques and technologies with new forms of integration to reveal insights from datasets that are diverse, complex, and of a massive scale.
10 The United Nations Sustainable Development Goals (SDGs) or Global Goals are a collection of 17 interlinked goals, set in 2015, designed to be a "blueprint to achieve a better and more sustainable future for all". They address the global challenges we face, including poverty, inequality, climate change, environmental degradation, peace, and justice.
Conclusion
Quantitative investing is alive and well! Such an approach is definitely appropriate for ESG investing, yet quant must learn to speak ESG.
The amount of data keeps growing and we are getting more data history by the day. However, one must bear in mind that quantitative techniques are only a means to an end; the end is about making informed investment management decisions in line with investors’ sustainability values and risk tolerance, and in fine produce financial returns.
I cannot radically oppose quantitative investing and fundamental analysis because, whether they admit it or not, quantitative managers often leave room for fundamental analysis or, what they sometimes call “judgmental overlays”. The amount of room varies from one manager to another. However, leaving no room at all amounts to be falling in the category of ‘strictly computer-driven’ managers, which I described earlier.
Quantitative models rely on foundational assumptions set by investment professionals; they also cannot account for all possible parameters and scenarios. For those reasons, an investment manager must often introduce a healthy dose of fundamental analysis in the processes in order to successfully achieve its goals.
Finally, it should be emphasised that a quantitative approach enables the managers to run iterative processes that continuously help improve and refine the models, thereby adapting to different market cycles.
Habib Moudachirou is CIO of V-Square Quantitative Management.
DISCLOSURES
This information is intended for educational purposes and should not be considered a recommendation to buy or sell a particular security.
The information in this document is provided in good faith without any warranty and is intended for the recipient's background information only. It does not constitute investment advice, recommendation, or an offer of any services or products for sale and is not intended to provide a sufficient basis on which to make an investment decision. It is the responsibility of any persons wishing to make a purchase to inform themselves of and observe all applicable laws and regulations. Unauthorized copying, reproducing, duplicating, or transmitting of this document are strictly prohibited. V-Square Quantitative Management accepts no responsibility for loss arising from the use of the information contained herein.
© 2020 V-Square Quantitative Management LLC. All rights reserved